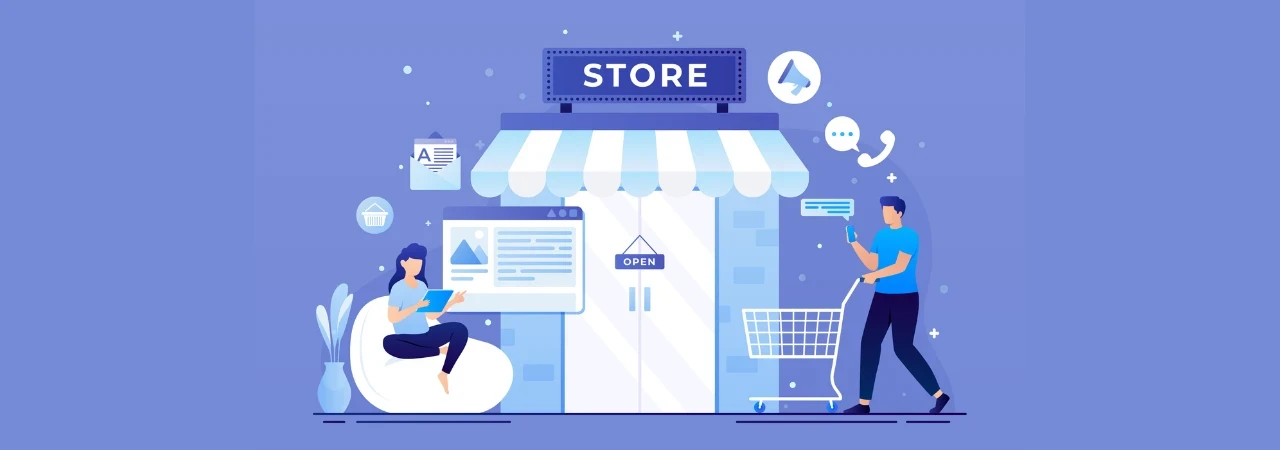
Machine Learning is probably one of the most commonly discussed topics in the world of technology. In fact, most technology companies from start-ups to MNCs have today invested in Artificial Intelligence (AI) and Machine Learning (ML) projects.
In the past few years, sources of data capture have evolved extensively to give businesses access to enormous volumes of data. Companies no longer limit themselves to surveys, questionnaires, and other traditional forms of data collection to gain insights into consumer behavior. Smartphones, cookies, drones, sensors, and cameras are the modern form of data collection.
What is Machine Learning?
The term “Machine Learning” was first defined by Arthur Samuel in 1959. According to him, “Machine Learning is the sub-field of computer science that gives computers the ability to learn without being explicitly programmed.”
In simple words, Machine Learning is a set of techniques used to program computers and equip them to make decisions automatically. Decisions are arrived at by detecting (or learning) patterns in past data and generalizing such patterns on future data. A simple example will be mail spam filters. Mail services such as Google, Yahoo, and Hotmail use Machine Learning to detect whether an email falls into the spam category or not.
Consumer behaviour:
The ever-changing market trends make it difficult for businesses to understand consumer behavior accurately. A detailed insight is needed to understand the buying and consumption pattern of consumers, needs/wants/motives to buy/sell/use a particular product, and capture which type/category of customers is based on demographic variables. Techniques like Machine Learning can bring the desired results-oriented towards user engagement and revenue generation. Marketing departments are more than ever engaged in discovering newer ways of making advertising and branding campaigns effective.
Consumer decisions & Machine Learning:
The Consumer Decision Processes (also known as Buyer Decision Process) refers to the decision-making stages that a consumer undergoes before, during, and after purchasing a product or service.
● Need Recognition: This is recognized as the first and most crucial step in the process. Need in fact, is the catalyst that triggers the buying decision of consumers.
● Research: After the consumer has developed a want or a need, they initiate information search about the different alternatives available in the market
● Evaluation of Alternatives and Purchase : An individual, after gathering relevant information, tries to choose the best option available, as per their needs, tastes, and budget. Finally, they purchase the product
● Post-Purchase Evaluation: This refers to a customer’s analysis of whether the product was useful to them or not.
Machine learning and data analytics play a vital role in the consumer buying behavior scenario.
1) Consumer online search:
Google and its competitors are constantly improving on what the search engine understands. Whenever we search on Google, the search engine watches how you respond to results. If you click the top result and stay on that page, it assumes that we received the right information and that the search was a success. If you click on the second page (of results), or type in a new search string without clicking any of the results, the search engine will learn from that mistake and use it to deliver better results in the future.
2) Marketing personalization:
Most of us had the experience of visiting a physical store, looking at a product, and not buying it. We then visit the web for the same product. This is something that eCommerce companies can use increasingly for personalization. This can be done through promotional emails. After analyzing consumer search patterns, such companies can also target people through coupons, offers, and product recommendations which ultimately culminate in a sale.
3) Learning from past behavior of consumers:
A major advantage of Machine Learning is that we can learn from past predictions and outcomes, and continually improve predictions based on new and different data.
4) Recommendation systems:
Recommendation systems are used by eCommerce sites to suggest relevant products to their customers. Types of recommendations include suggesting products to the consumer, which products others bought, summarization of community opinion, and providing customer reviews.
5) Product matching to preferences:
Machine learning coupled with data analytics can present a crystal-clear picture of consumer behavior. Algorithms play a crucial role when it comes to determining price points that can help leading brands position products to the right target market.
6) “Up-sell” and “Cross-sell”:
Cross-selling is when you recommend that your customer buy a product that compliments an existing purchase from a different category or vendor. Such recommendations are a great way to help visitors discover other products on your online store and deliver a personalized shopping experience to them. Studies show that product recommendations can increase average order value by up to 15-30% and boost conversion rates by 10 – 30%. Effective Cross-selling and Up-selling can increase total orders, conversion rates, exposure to higher-margin items, selecting the bestseller, and also improve customer retention rates, which means higher lifetime value customers.
Final word:
Machine Learning is a great way to gain insights into customer behavior and give them what they want well ahead. It ensures their loyalty when combined with the right price, quality and innovation. Shopping behavior is now being tracked relentlessly to make the most out of customer dollars.
A flip side of such learning would be the privacy concerns of consumers. This is not an ill-founded fear, as no one likes being targeted for sales, particularly when it is known that we are being tracked. Retailers have to be sensitive to consumer preferences for privacy when utilizing new-age technologies that aid decision-making.